Joel C. Miller
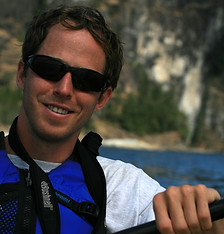
Probability Generating Functions
A Probability Generating Function (PGF) is a function of the form
p_0 x^0 + p_1 x^1 + p_2 x^2 + . . . (I hope to sort out math text better in the future)
​
where we can think of p_n as the probability of n events happening.
​
These have many applications in infectious disease, where usually we interpret the event as being a transmission.
A few publications
Here are a few examples of where I have used PGFs in my research.
PGF Methodology
I wrote a 60+ page paper describing how to apply PGFs to infectious disease modelling. The paper is really a mini-book, complete with exercises and appendics. It was initially intended to be a short 4-page internal note at the Institute for Disease Modeling (where I was at the time) explaining how to use PGFs to predict epidemic probability. But it kept expanding.
Edge-based Compartmental Models
The EBCM (edge-based compartmental model) approach that I developed based on a paper by Erik Volz uses PGFs to reduce the number of equations needed to model a heterogeneous population to just a handful. Without PGFs,, the number of equations we would need would be proportional to the number of degrees observed in the population.
​
On the 'publications' page, references 23, 24, 26, 32, and 33 develop this. I recommend starting with reference 26.
Understanding Super-Spreading
Early in an epidemic, there is a big difference between an epidemic where every individual causes 2 infections or where 1% would cause 200 infections and 99% would cause none, even though in both cases the average is the same. An epidemic is guaranteed from one infection in the first case, and unlikely in the second.
​
PGFs allow us to study the possible different outcomes. We applied this to understanding the early spread of COVID-19